About Me
Hi! I'm Alex Mara an AI researcher and entrepreneur based in Ghent, Belgium. Originally from Romania, I have spent most of my adult life in various European countries including Spain, Italy, Finland, Germany and Belgium. I am deeply passionate about AI and the myriad of opportunities it can bring us, so I built my entire career around it. To offset this nerdy part of my life, I also practice many sports such as callisthenics, bouldering and mountain biking. I am also a huge fan of videogames both modern and old-school (P.S.: try searching for a non-existent subdomain on my website, like this one :P). In what follows, you can learn more about myself, my interests, publications, projects, teaching... just keep reading!
Currently, I am one of the Co-Founders and CEO of Nobl.ai, a tech startup focused on delivering trustworthy AI for the labour market. We provide state-of-the-art recommender systems that match candidates and jobs, mapping (normalization) of job descriptions and courses into standard (ISCO, ESCO, O*Net, ...) and proprietary taxonomies, career coaching, and much more! If you are interested in learning more, drop me a message!
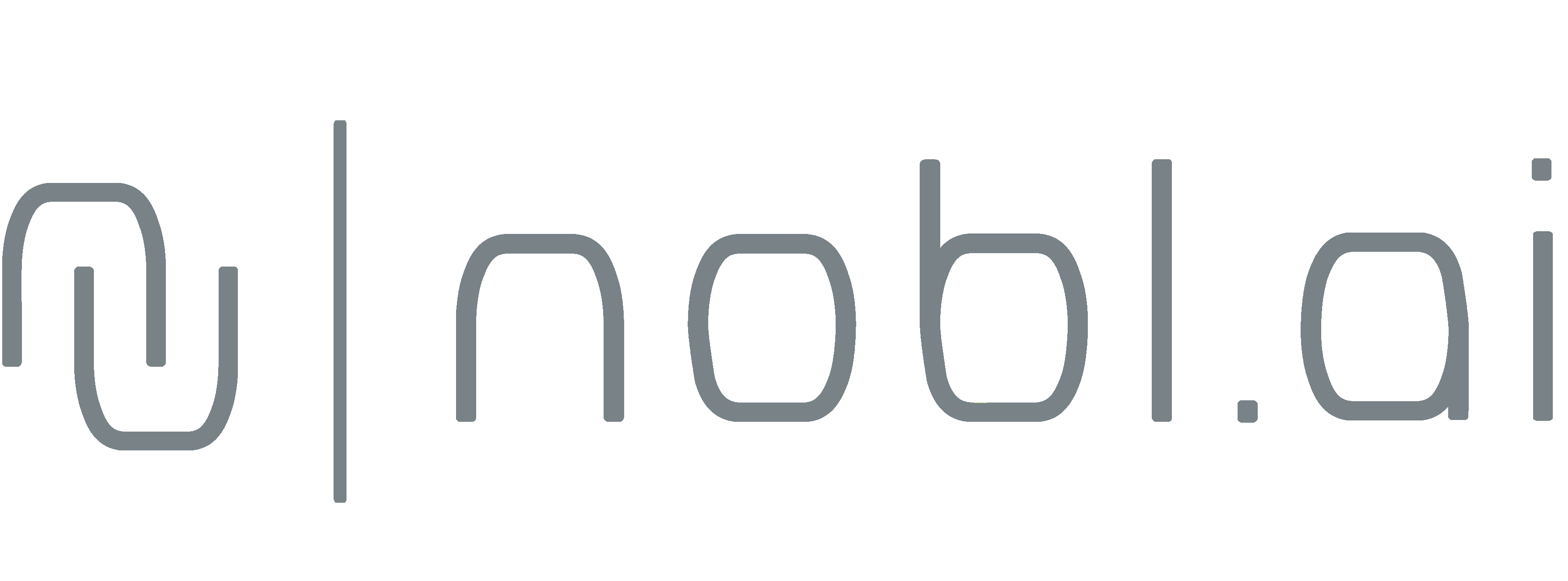
I also hold a part-time appointment as a Postdoctoral Researcher in the Artificial Intelligence and Data Analytics (AIDA) group at Ghent University led by Prof. Tijl De Bie. My research interests revolve around recommender systems and representation learning as well as their applications to the labour market. Over the past decade, I have built an international academic career in AI and data mining obtaining a PhD from Ghent University in Belgium, an MSc. (with honours) from Aalto University in Finland and a B.Eng. (first class honours) from the Technical University of Madrid in Spain. You can check out my full academic CV here.
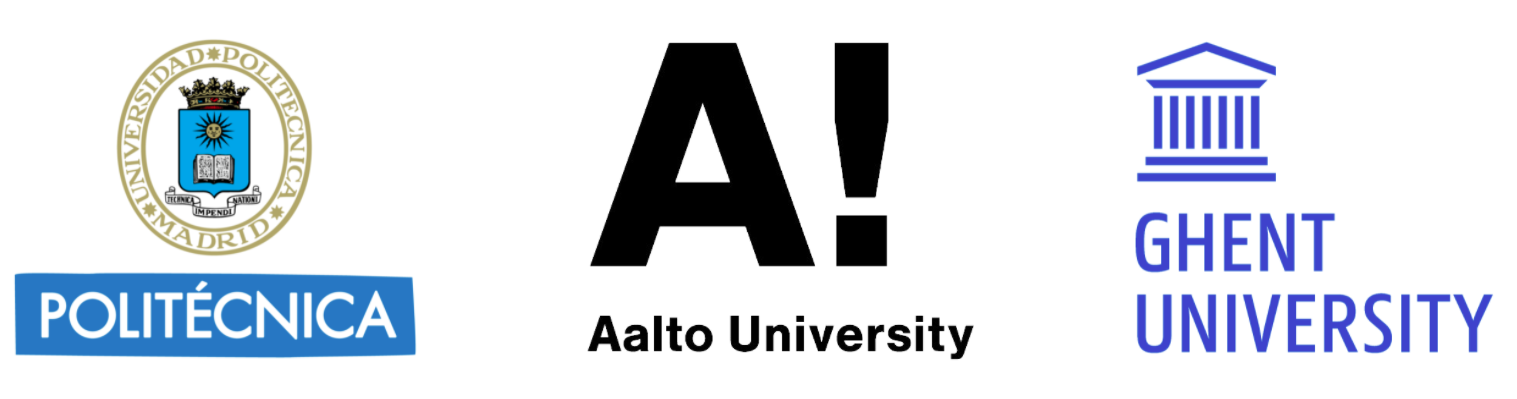
Below you can find some of my main publications, but for an always up-to-date list, you can take a look at my Google Scholar profile. The code of my open-source projects can be found on GitHub here and here.
Research Interests
Representation Learning
Dimensionality Reduction
Recommender Systems
Graph Semi-supervised Learning
Big Data Analytics
Distributed Computing
Experimental Design & Evaluation
Extreme multi-label classification
AI for HR and the labour market
Publications
Dissertations
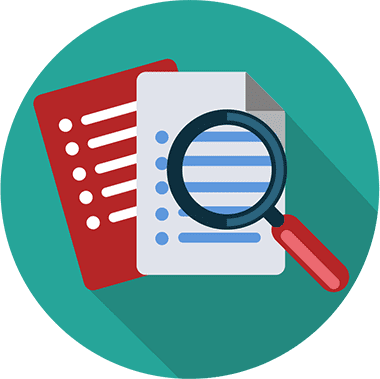
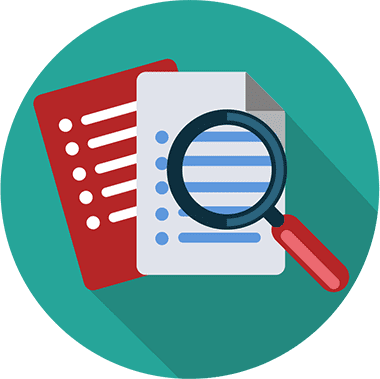
Journal Articles
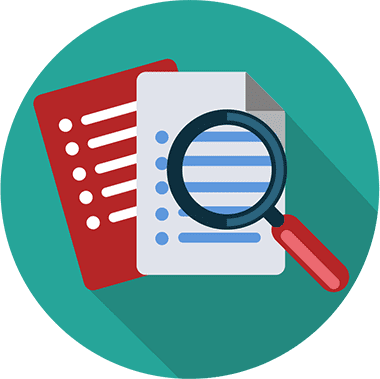
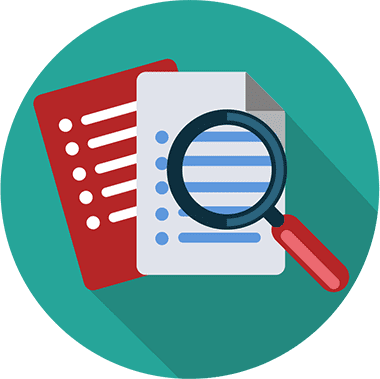
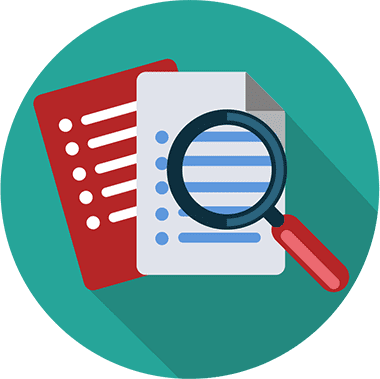
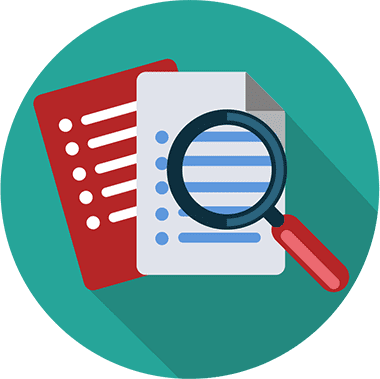
Conference Proceedings
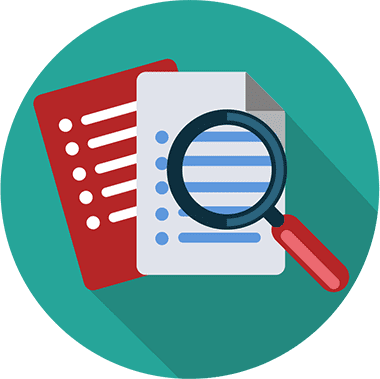
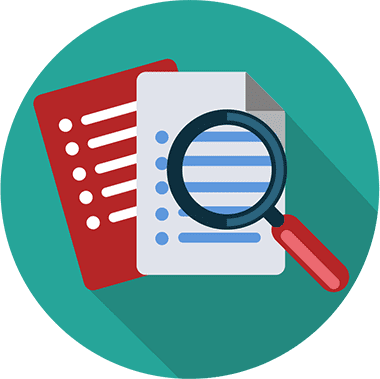
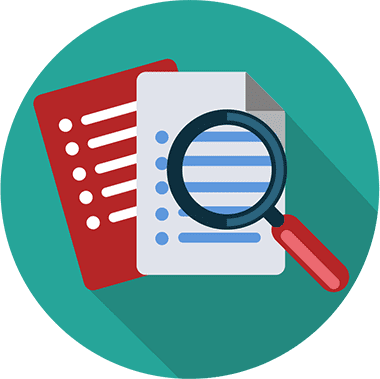
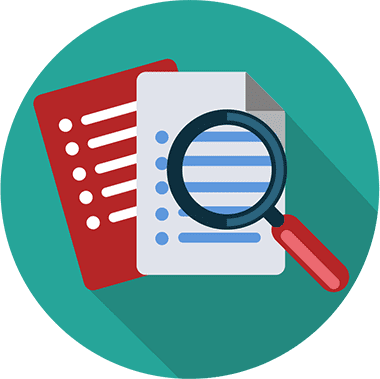
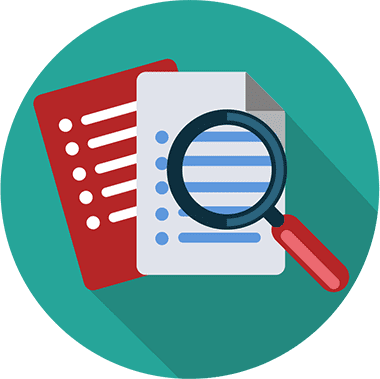
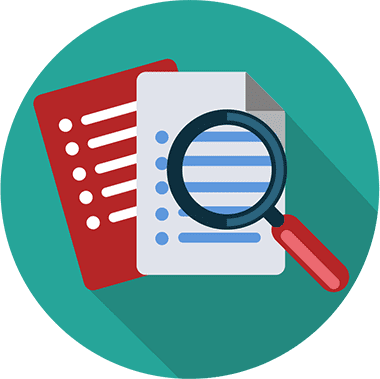
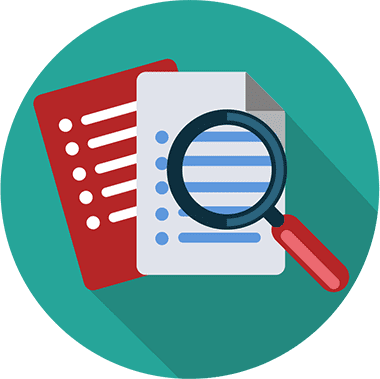
Teaching Experience
- Teaching Assistant: AI Research Seminar, Ghent University, 2021/2022
- Teaching Assistant: Big Data Science, Ghent University, 2018/2019/2020
- Teaching Assistant: Machine Learning Basic Principles, Aalto University, 2016
- Teaching Assistant: Convex Optimization for Big Data, Aalto University, 2015
- Teaching Assistant: Mathematical Analysis, Technical University of Madrid, 2011/2012/2013
Other Activities
- Virtual conference chair: ECMLPKDD'20
- Area chair: ICDM'23, ICDM'24
- Program committee member: ICDM, ECML-PKDD
- Reviewer: Springer Nature, IEEE TNNLS, IEEE TSP, Information Processing and Management, Big Data, SoftwareX, MDPI Applied Sciences, MDPI Mathematics
- Group Lead: Slush Music construction team, 2017
- Volunteer: Slush event help team, 2016
- Volunteer: ESN Aalto, 2016-2017